Introduction
In the modern era, network traffic analysis has evolved from a niche IT function to a critical cybersecurity and network management component. As organizations increasingly rely on digital infrastructure, the complexity and volume of network traffic have grown exponentially. Traditional network analysis tools, while still valuable, often struggle to keep up with this new reality. Enter AI-based network traffic analysis tools—advanced solutions designed to enhance visibility, improve security, and optimize network performance.
This article comprehensively reviews AI-based network traffic analysis tools, exploring their features, benefits, and potential drawbacks. We will also examine critical market players and provide insights into how these tools can be effectively integrated into your organization’s network management strategy.
The Evolution of Network Traffic Analysis
Traditional Methods
Historically, network traffic analysis relied on signature-based detection, rule-based systems, and manual inspection. Tools like Wireshark, Snort, and NetFlow provided valuable insights by capturing and analyzing packets, detecting known threats, and monitoring network performance. However, these methods have limitations, mainly when dealing with zero-day threats, encrypted traffic, or sophisticated attacks that evade signature detection.
The Advent of AI in Network Traffic Analysis
Artificial Intelligence (AI) has transformed many aspects of IT, and network traffic analysis is no exception. By leveraging machine learning (ML) algorithms, AI-based tools can analyze vast network data, identify patterns, and detect anomalies that might indicate malicious activity. These tools can adapt to changing network conditions and emerging threats, providing agility and intelligence that traditional methods cannot match.
Key Features of AI-Based Network Traffic Analysis Tools
AI-based network traffic analysis tools come with several key features that set them apart from traditional solutions:
1. Anomaly Detection
One of AI’s most significant advantages is its real-time detection of anomalies. Machine learning models can be trained to recognize “normal” network behaviour. When the network deviates from this baseline, the AI system can flag potential issues, whether signs of a cyberattack, a network misconfiguration, or an unusual spike in traffic.
2. Automated Threat Detection
AI-powered tools excel at identifying threats without requiring pre-defined rules or signatures. By analysing network traffic behaviour, they can detect unknown malware, phishing attempts, and other sophisticated attacks. This capability is crucial in the modern threat landscape, where new types of attacks are constantly emerging.
3. Behavioral Analysis
AI tools can monitor and analyze user behaviour on the network. By understanding the typical actions of users and devices, these tools can spot deviations that might indicate compromised accounts or insider threats. This analysis goes beyond traditional network traffic monitoring, providing deeper insights into potential security risks.
4. Scalability
AI-based tools are designed to handle large volumes of data, making them ideal for organizations with extensive networks. They can process and analyze traffic across multiple devices, applications, and locations, providing a comprehensive view of network activity.
5. Automated Response
Some AI-based tools are equipped with automated response capabilities. When a threat is detected, these tools can take immediate action, such as blocking malicious traffic, isolating affected devices, or alerting security teams. This rapid response can significantly reduce the time it takes to contain and mitigate threats.
Benefits of AI-Based Network Traffic Analysis Tools
The integration of AI into network traffic analysis offers numerous benefits:
1. Enhanced Security
AI-based tools significantly enhance network security by detecting threats that traditional methods might miss. Their ability to identify zero-day exploits, advanced persistent threats (APTs), and other sophisticated attacks makes them a critical component of a robust cybersecurity strategy.
2. Reduced False Positives
Traditional network analysis tools often generate false positives, leading to alert fatigue among security teams. With their advanced pattern recognition capabilities, AI-based tools can reduce false positives by accurately distinguishing between benign anomalies and genuine threats.
3. Improved Network Performance
AI tools can optimize network performance by identifying and addressing issues before they escalate. For example, they can detect bottlenecks, optimize bandwidth usage, and ensure critical applications have the resources they need to function smoothly.
4. Cost Efficiency
While AI-based tools can be an investment, they often lead to cost savings in the long run. By automating many aspects of network monitoring and threat detection, these tools can reduce the need for manual intervention, lower the risk of costly breaches, and optimize network resources.
5. Proactive Threat Hunting
AI-based tools empower organizations to take a proactive approach to cybersecurity. Instead of simply reacting to incidents after they occur, these tools can identify potential threats before they materialize, allowing security teams to take preemptive action.
Challenges and Limitations
Despite their many advantages, AI-based network traffic analysis tools are not without challenges:
1. Complexity
Implementing AI-based tools can be complex, requiring a deep understanding of machine learning models and algorithms. Organizations may need to invest in training or hire specialized staff to manage these tools effectively.
2. Data Privacy Concerns
AI-based tools require access to large amounts of network data to function effectively. This can raise privacy concerns, mainly if sensitive information is being analyzed. Organizations must comply with data protection regulations and implement appropriate safeguards.
3. Cost
While AI tools can lead to cost savings in the long term, the initial investment can be significant. Small and medium-sized enterprises (SMEs) may struggle to justify the expense, especially if they do not have a dedicated cybersecurity budget.
4. Dependence on Data Quality
The effectiveness of AI-based tools depends heavily on the quality of the data they analyze. Poor-quality data can lead to inaccurate predictions and missed threats. Organizations must ensure that their data is clean, comprehensive, and up-to-date.
5. False Negatives
While AI tools can reduce false positives, they are not infallible. There is always a risk of false negatives—when a threat goes undetected. Continuous monitoring and updates are necessary to minimize this risk.
Market Overview: Leading AI-Based Network Traffic Analysis Tools
Several companies have emerged as leaders in AI-based network traffic analysis. Below, we review some of the most prominent tools available today.
1. Darktrace
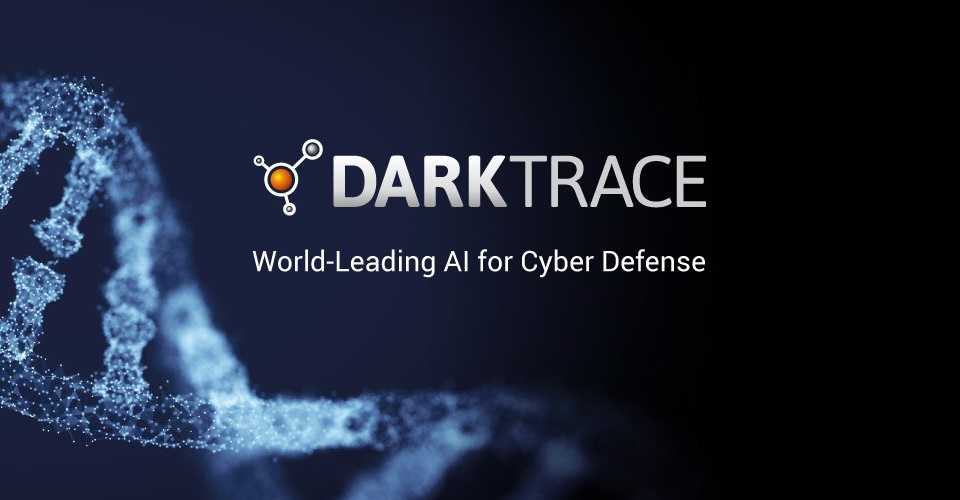
Darktrace is one of the most well-known names in AI-based cybersecurity. Its Enterprise Immune System uses unsupervised machine learning to detect and respond to threats in real time. Darktrace’s AI is modelled after the human immune system, allowing it to identify and neutralize threats autonomously. The platform also offers the Antigena module, which can take automated actions to contain threats.
Pros:
- Real-time threat detection and response.
- Autonomous threat mitigation with minimal human intervention.
- Adaptive learning that improves over time.
Cons:
- High cost, making it less accessible for SMEs.
- Some users report a steep learning curve.
2. Cisco Secure Network Analytics (formerly Stealthwatch)
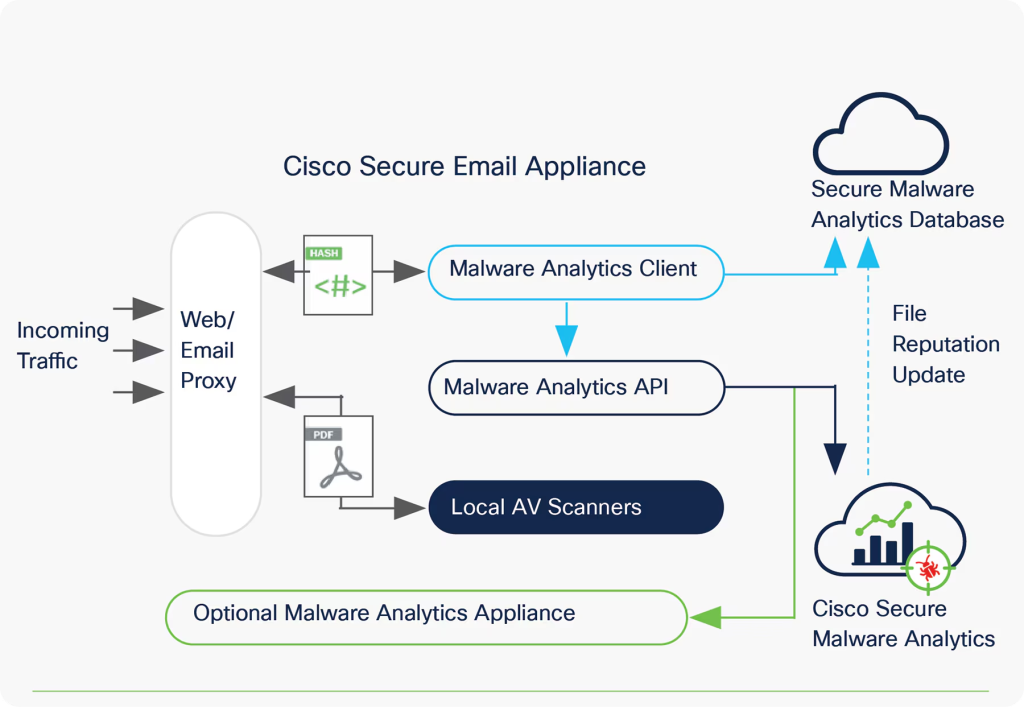
Cisco’s Secure Network Analytics uses machine learning to provide comprehensive visibility into network traffic. The tool can detect threats, from insider attacks to advanced malware, and integrates seamlessly with other Cisco security products.
Pros:
- Strong integration with the broader Cisco ecosystem.
- Effective at detecting insider threats and lateral movement.
- Scalable for large enterprise networks.
Cons:
- It may require significant configuration and tuning.
- It can be expensive, especially for smaller organizations.
3. Vectra AI
Vectra AI’s Cognito platform detects and responds to cyberattacks in real time. It uses AI to analyze network metadata and detect hidden threats, such as command-and-control communications and lateral movement. Vectra AI also offers a cloud-based solution for securing cloud environments.
Pros:
- Effective at detecting advanced threats and APTs.
- Provides clear, actionable insights and alerts.
- Strong focus on cloud security.
Cons:
- Requires deep integration into existing network infrastructure.
- Higher cost compared to some competitors.
4. ExtraHop
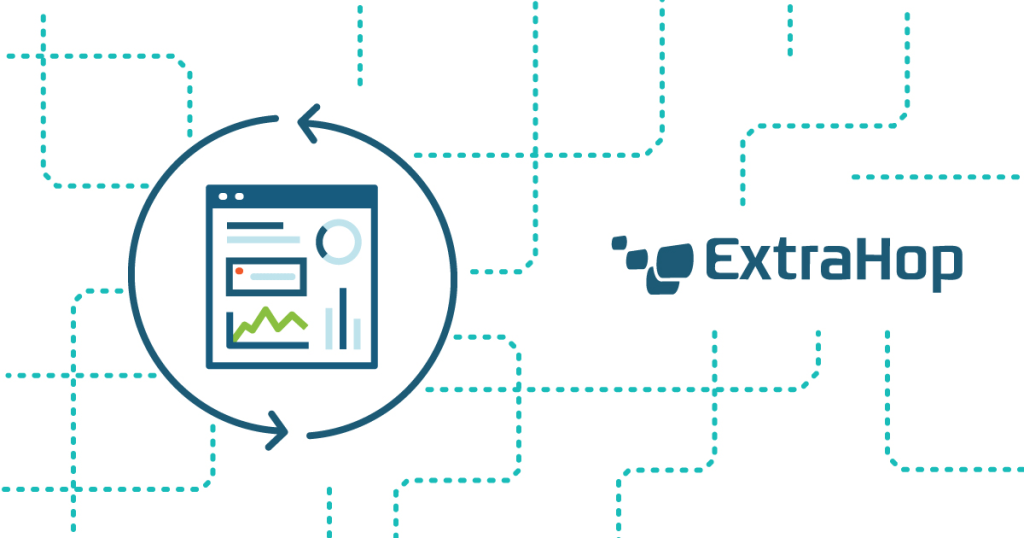
ExtraHop offers an AI-powered network detection and response (NDR) platform that provides complete visibility into network traffic. The tool uses machine learning to detect anomalies and potential threats across on-premises, cloud, and hybrid environments.
Pros:
- Comprehensive visibility across various environments.
- Effective at detecting encrypted threats.
- Strong focus on performance monitoring in addition to security.
Cons:
- It can be complex to deploy and manage.
- It may require a significant initial investment.
5. Plixer Scrutinizer
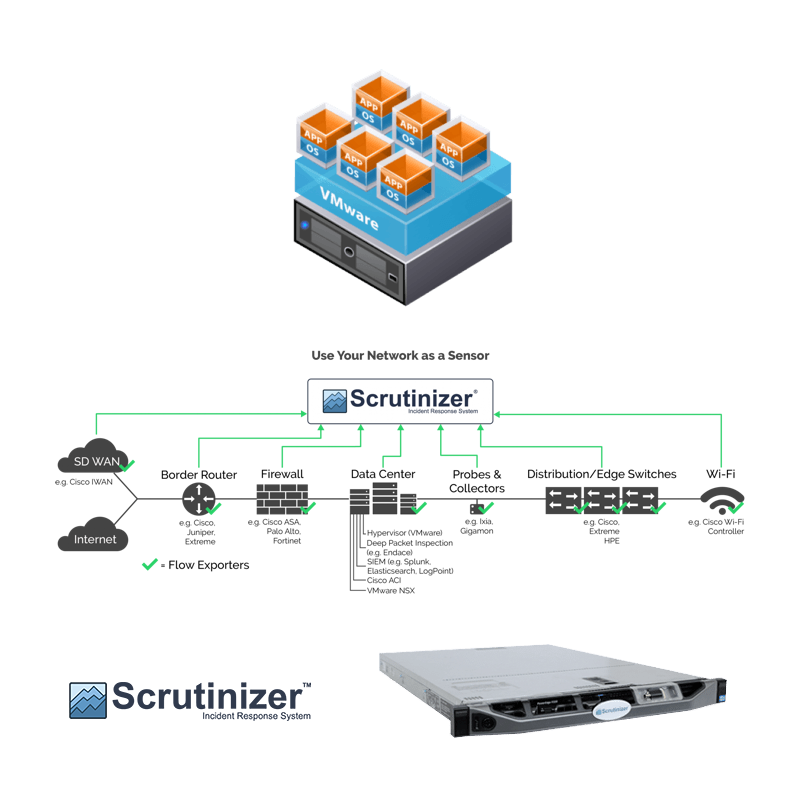
Plixer Scrutinizer combines flow and machine learning to deliver network traffic analysis and threat detection. It offers detailed visibility into network performance and security, making it a versatile tool for network management and cybersecurity.
Pros:
- Combines security and performance monitoring.
- Cost-effective compared to some high-end solutions.
- User-friendly interface and reporting.
Cons:
- Less advanced in threat detection compared to specialized AI tools.
- It may require integration with other security solutions for comprehensive coverage.
Choosing the Right AI-Based Network Traffic Analysis Tool
Selecting the proper AI-based network traffic analysis tool depends on several factors, including the size of your organization, the complexity of your network, and your specific security needs. Here are some considerations to keep in mind:
1. Budget
AI-based tools vary significantly in cost. While some high-end solutions offer unparalleled features, they may be out of reach for smaller organizations. Balancing the need for advanced security with the available budget is essential.
2. Scalability
Consider how well the tool can scale with your organization. If you’re a growing company, you’ll need a solution to handle increasing network traffic without compromising performance.
3. Integration
Ensure that the tool can integrate seamlessly with your existing IT
infrastructure. This includes compatibility with other security tools, network devices, and cloud environments.
4. Ease of Use
AI-based tools can be complex, so choosing a solution your team can manage effectively is essential. Look for tools with intuitive interfaces, comprehensive documentation, and strong customer support.
5. Specific Features
Identify the specific features that are most important to your organization. For example, choose a tool that excels if you prioritize real-time threat detection. If you’re focused on network performance, look for a solution that offers robust monitoring capabilities.
Implementation Best Practices
Implementing an AI-based network traffic analysis tool requires careful planning and execution. Here are some best practices to ensure a successful deployment:
1. Define Your Objectives
Before implementing the tool, clearly define what you hope to achieve. Are you primarily focused on improving security, enhancing network performance, or both? Your objectives will guide the selection process and inform how you configure and use the tool.
2. Start with a Pilot Program
Begin with a pilot program to test the tool in a controlled environment. This allows you to evaluate its effectiveness and make necessary adjustments before a full-scale deployment.
3. Monitor and Adjust
AI-based tools often require ongoing monitoring and adjustments to perform optimally. Regularly review the tool’s performance, update its machine learning models, and refine its configurations based on your network’s evolving needs.
4. Train Your Team
Ensure your IT and security teams are adequately trained to use the tool. This includes understanding its features, interpreting its alerts, and responding to potential threats.
5. Stay Up-to-Date
Cyber threats are constantly evolving, and so should your tools. Keep your AI-based network traffic analysis tool up-to-date with the latest threat intelligence and software updates.
Conclusion
AI-based network traffic analysis tools significantly advance cybersecurity and network management. By leveraging the power of machine learning, these tools can detect and respond to threats more effectively than traditional methods, offering enhanced security, improved network performance, and cost efficiencies.
However, these tools are not a one-size-fits-all solution. Organizations must consider their needs, resources, and network environments carefully when choosing and implementing an AI-based network traffic analysis tool. By following best practices and staying informed about the latest developments, you can harness AI’s full potential to protect and optimize your network in today’s increasingly complex digital landscape.
Links in this article may be affiliate links, meaning they could generate compensation to us without any additional cost to you should you choose to purchase a paid plan. These are products we have personally used and confidently endorse. It’s important to note that this website does not offer financial advice. You can review our affiliate disclosure in our privacy policy for more information.